Accelerate AI Literacy and Transformation
SmarterX is an AI research and consulting firm.
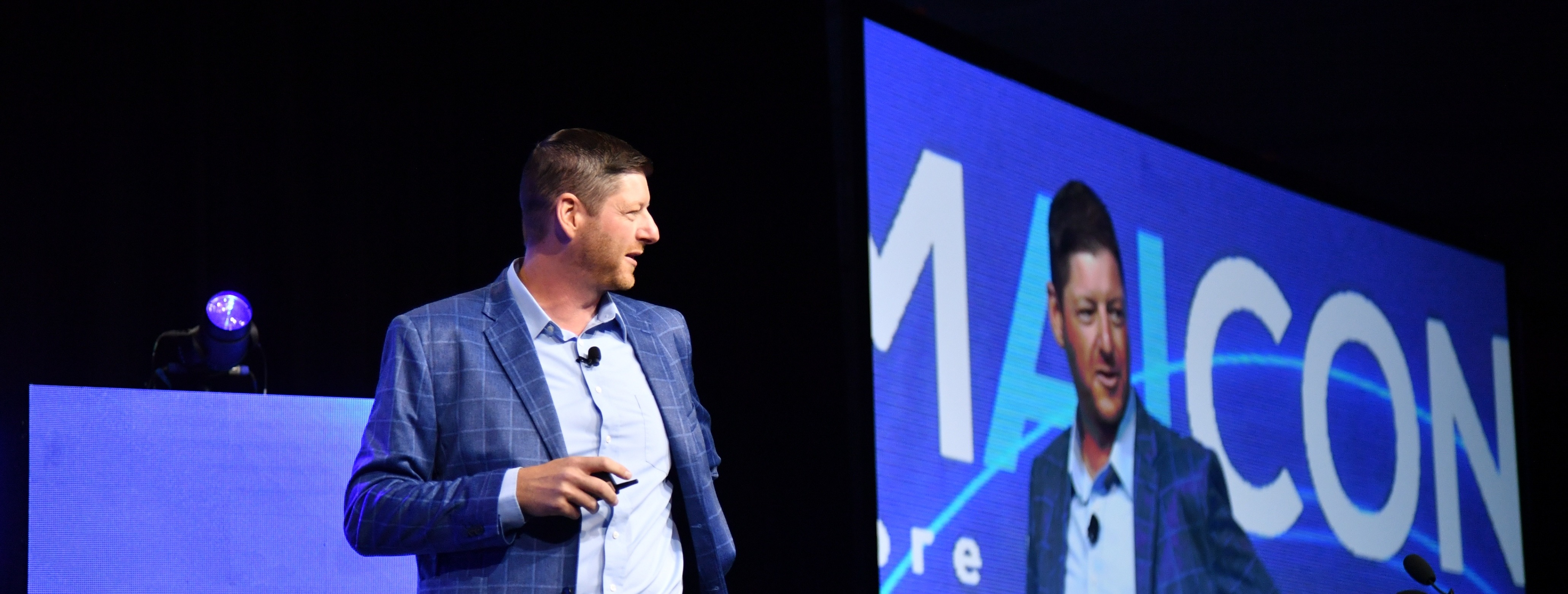
SmarterX was introduced by founder and CEO Paul Roetzer in 2022 as the consulting arm of Marketing AI Institute, and spun out as its own AI research and consulting firm in 2024.
SmarterX educates and empowers leaders to reimagine business models, reinvent industries, and rethink what’s possible.
Advisory engagements are led by Roetzer, who has 20+ years of consulting experience. During his 16 years as a marketing agency CEO and lead strategist, he advised more than 400 clients across dozens of industries.
SmarterX gives business leaders unparalleled access to the knowledge, resources, technologies, and strategies they need to build smarter, AI-forward businesses.
Learn more about SmarterX at www.SmarterX.ai.
Marketing AI Institute and SmarterX are sister companies owned and operated by SmarterX Ventures, LLC.